The Unbeatable Combo of AI and Edge Computing
As mobile devices and IoT applications continue to skyrocket, billions of new edge devices are being connected to the Internet and producing vast quantities of data. That's why amassing such huge data stores in the cloud uses up so much bandwidth and time due to the delay involved. Therefore, to fully unlock the potential of big data, it is crucial to push the boundaries of artificial intelligence (AI) to the network edge. One of the most important ideas in cutting-edge AI applications is edge AI.
Do you know
According to Grand View Research, the worldwide Edge computing industry will grow by 37.4% annually and reach $43.4 billion by 2027.
Answering questions like "How does Edge AI work?" "What kind of business benefits does it bring?" and "How can I get started with Edge AI?"
Find out here; let's start our voyage to the edge!
Edge AI is driven by Big Data and IoT
Now that we live in the Internet of Things (IoT) era, we must collect and analyze an enormous amount of data from our networked devices. As a result, a flood of new data is being produced in near real-time, necessitating the use of artificial intelligence systems to decipher it.
Edge AI, in its simplest form, combines two technologies: edge computing and AI.
Edge Computing
Various methods that relocate data collection, analysis, and processing to the network's periphery constitute what is known as "edge computing." Computing resources and data are thus situated close to the data collection point. A mobile phone, Internet of Things (IoT) device, autonomous vehicle, or cell tower might serve the purpose.
Artificial Intelligence
Artificial intelligence refers to computer systems that perform tasks typically associated with human intelligence, such as language comprehension and problem-solving. One definition of artificial intelligence is the combination of advanced analytics (often based on machine learning) with robotic process automation (RPA).
All current uses of AI fall under this pragmatic notion.
Read the article on Commercializing Enterprise Automation through Robotic Process Automation as a Service.
The use of Edge AI to power Machine Learning in IoT Edge Devices
Using AI algorithms to interpret data locally on hardware, or "edge devices," Edge AI combines edge computing with artificial intelligence. As an on-device AI, it allows for taking advantage of low-latency, high-privacy, more robust, and efficiently used network capacity. Modern developments in machine learning (ML), neural network acceleration, and reduction are driving the demand for Edge AI. New, resilient, and scalable artificial intelligence (AI) systems in various fields are now possible because of ML edge computing. Every aspect of this field is cutting-edge and developing rapidly. Future AI development is anticipated to be led by edge AI, which brings AI capabilities closer to the real world.
Benefits of Edge AI
To get around the inherent issues of the traditional cloud, like high latency and a lack of security, edge computing allows AI processing duties to be moved from the cloud to near-the-end devices. Therefore, new possibilities for AI applications with new products and services become available when AI computations are transferred to the network edge.
1. Reduced data transmission
The edge device performs data processing, with only a minimal amount of the result forwarded to the cloud. The traffic in the core network can be lowered, and the connection bandwidth between a small cell and the core network can be enhanced to avoid bottlenecks.
2. Real-time computing speed
Edge Computing's ability to process data in real-time is a major benefit. The closer the edge device is located to the source data, the less time it takes to process the data in real-time, increasing its usefulness. It's useful for services and applications that can't afford to wait, such as telemedicine, tactile Internet, autonomous vehicles, and crash avoidance systems. In real-time, edge servers can offer various services such as decision support, decision-making, and data analysis.
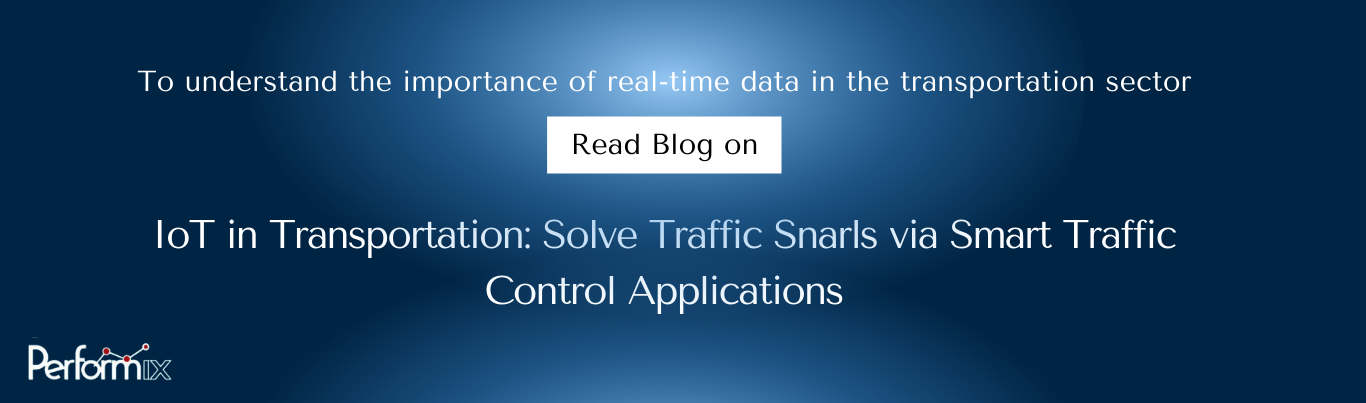
3. Scalability
According to IDC's forecasts, by 2025, 41.6 billion IoT devices will be in use, creating 79.4 zettabytes of data. As data volumes increase, there is a pressing need for novel, effective analysis, and processing methods.
Most data processing can be done locally, at the edge, so transferring data or using a centralized service doesn't slow things down. In most cases involving artificial intelligence at the edge, a considerable amount of data is concerned. Transferring the data to a cloud service is not an option if you need to simultaneously process video picture data from hundreds or thousands of sources.
4. Security and privacy
Due to the security risks associated with sending sensitive user data via a network, many organizations opt to deploy AI systems in the network's periphery. When using edge computing, users can be sure their data is safe on-premises (on-device machine learning). Edge devices can scrub data of personally identifiable information before sending it to a remote server, making the process safer and more private for end users.
5. Excellent Availability
Edge AI is more secure since it is distributed and can function offline, delivering temporary services even when the network is down or under assault. Since mission-critical or production-grade AI systems require much higher availability and general robustness, their deployment to the edge is the best option.
6. Economic Benefit
By offloading AI processing to the edge, only the most useful data is delivered to the cloud, thus reducing data transfer costs. Small devices near the edge have become more computationally capable, following Moore's Law, while providing and storing massive amounts of data remains costly. Edge-based ML, in general, overcomes the inherent limits of cloud computing to allow for real-time data processing and decision-making. Edge ML may soon be the only practical approach for businesses to utilize AI in products and services in light of the increasing importance of data privacy and regulatory changes like GDPR.
Applications of Edge AI
Edge AI can now power scalable, mission-critical, and secure AI applications. Since Edge AI is still in its infancy, we can anticipate that it will see widespread use in various fields soon.
- Smart AI Vision's computer vision applications, such as real-time video analytics, can power AI vision systems in more than one industry. Intel created specialized co-processors, Visual Processing Units, for high-performance computer vision applications on edge devices.
- Applications for Smart Factories, such as intelligent machinery, aim to increase safety and production. Using a remote platform, for instance, personnel can handle heavy machinery safely and comfortably, particularly in inaccessible and hazardous areas.
- Smart transportation technologies allow drivers to exchange or gather information from traffic information centers in real-time to avoid collisions by avoiding vehicles that are in danger or stopping abruptly. In addition, autonomous vehicles can detect their surroundings and navigate safely on their own.
- Applications of Smart Energy- Networked wind farms- A study compared the data administration and processing costs of a remote wind farm utilizing a cloud-only system vs. a mixed edge-cloud solution. The edge-cloud system was 36% less expensive than the cloud-only system, but the amount of data needed to be transported decreased by 96%. The wind farm uses several data-generating sensors and devices, including video surveillance cameras, security sensors, staff access sensors, and sensors on wind turbines.
- Virtual reality, augmented reality, and mixed reality applications include streaming video content to virtual reality glasses. The size of these glasses can be reduced by offloading processing from these glasses to edge servers near the end device. Microsoft, for instance, just released HoloLens, a holographic computer integrated into a head-mounted display for augmented reality experiences. Microsoft intends to develop HoloLens tools for standard computing, data processing, medical imaging, and cutting-edge gaming.
- Healthcare applications such as remote surgery, diagnostics, and monitoring of patient vital signs rely heavily on edge devices that execute AI at the edge. Doctors can utilize a remote platform to manipulate surgical instruments from a safe and comfortable distance.
Why start with Edge AI?
Massive volumes of IoT-generated data
IoT and sensor technologies generate such voluminous amounts of data that their collection can be difficult or impossible. For example, the latest Airbus A350 aircraft have 50,000 sensors that capture 2.5 gigabytes of daily data. This is more data than WalMart's entire Teradata data warehouse in 1992.
Data is meaningless if it is separated from its source and needs metadata explaining its significance. Therefore, more than just retrieving data is required. The most vital information is stored in a data warehouse in the cloud or a data center. Locally analyzing vast amounts of sensor data and automating operational choices are also possible. To summarize, only Edge AI makes it feasible to utilize the much-hyped IoT data fully.
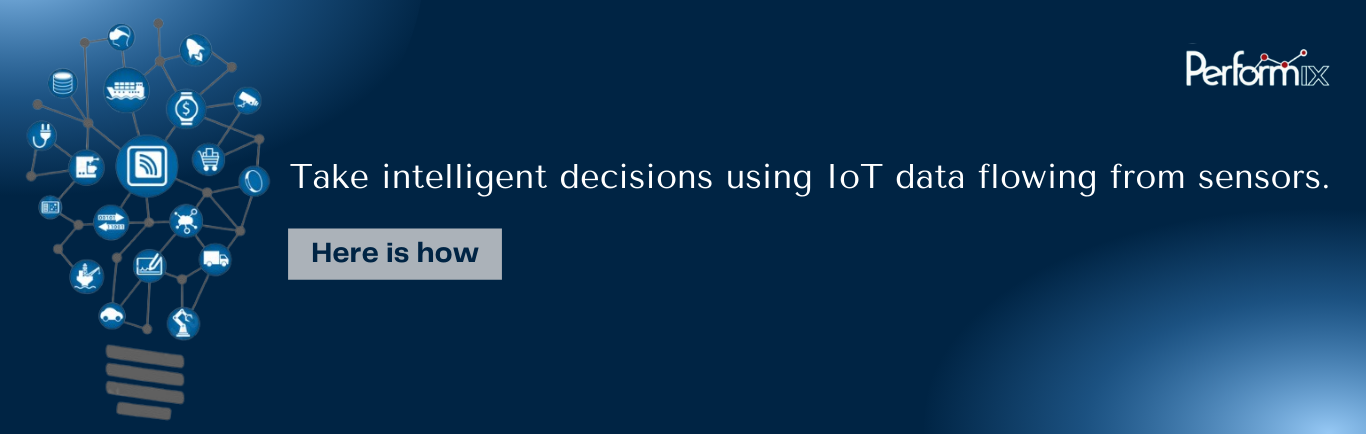
Expansion of 5G
5G networks allow for the capture of massive and rapid data streams. The building of 5G networks begins gradually, and initially, they will be deployed in heavily populated areas and on a relatively local scale. The usefulness of Edge AI technology improves when these data streams are utilized and analyzed as close as feasible to devices linked to the 5G network.
Edge computing is required for real-world applications of artificial intelligence because the standard concept of cloud computing is not suited for AI applications that are computationally heavy and require vast volumes of data. Edge computing was developed to circumvent this limitation.