The manufacturing industry, from its inception with assembly lines to the current robotics revolution, is embracing generative AI to address contemporary challenges. Supply chain disruptions and labor shortages are pressing concerns, with potential losses of 45% of annual earnings over the next decade. However, generative AI presents a path forward with the potential to revolutionize processes and enhance productivity.
Do you know
The U.S. manufacturing generative AI market, valued at USD 70.88 million in 2022, is forecast to exhibit remarkable growth. Projections suggest it will surpass USD 2,193.49 million by 2032, with an impressive CAGR of 41% from 2023 to 2032.
Explore More
Here are the top five compelling use cases for manufacturers to leverage generative AI:
1. Machine-Generated Events Monitoring: Revolutionizing Predictive Maintenance
Predictive maintenance, a strategy endorsed by Deloitte, can increase productivity by 25%, reduce breakdowns by 70%, and lower maintenance costs by 25%. Generative AI is pivotal in transforming maintenance workflows. It interprets telemetry data from equipment, enabling manufacturers to anticipate and prevent failures. By recommending solutions and service plans in real-time, it minimizes unplanned downtime and enhances operational efficiency.
2. Customer Service Automation: Elevating After-Sales Support
With 80% of business buyers expecting real-time interaction, manufacturers use generative AI to automate and enhance customer service. From troubleshooting product issues to ordering replacement parts, this technology provides a personalized and efficient customer service experience. The result is accelerated time-to-resolution for everyday interactions, influencing customer loyalty.
3. Document Search and Synthesis: Simplifying Complexity
Generative AI simplifies the complexity of product and service manuals in manufacturing. By swiftly extracting and summarizing information, it empowers service technicians with easily digestible instructions. Moreover, it streamlines ordering and quoting processes, allowing sales teams to navigate complex data effortlessly, leading to quicker and more accurate customer quotes.
4. Product/Content Catalog Discovery: Smart Sales Recommendations
Leveraging generative AI, manufacturers gain an efficient method to match product requirements with specifications. AI-enabled sales applications provide recommendations based on historical sales data, master data, and real-time feedback. This enables manufacturers to optimize their product offerings and enhance the overall buying experience for customers.
5. Supply Chain Advisor: Navigating Disruptions with Data-Driven Insights
Navigating the repercussions of supply chain disruptions and responding to the imperative of responsible sourcing, generative AI emerges as a pivotal supply chain advisor. As a strategic guide, it provides comprehensive visibility into intricate networks, furnishing real-time insights and tailored recommendations for optimal supplier selection. Through analyzing critical factors like raw material availability, delivery schedules, and sustainability metrics, decision-makers are empowered to address challenges and make informed choices in real time adeptly.
Embracing generative AI in these critical areas holds the promise of reducing downtime, improving output, realizing cost savings, and ultimately enhancing end-user satisfaction. As 82% of organizations anticipate transformative effects, the manufacturing industry is poised for a new era of efficiency and innovation.
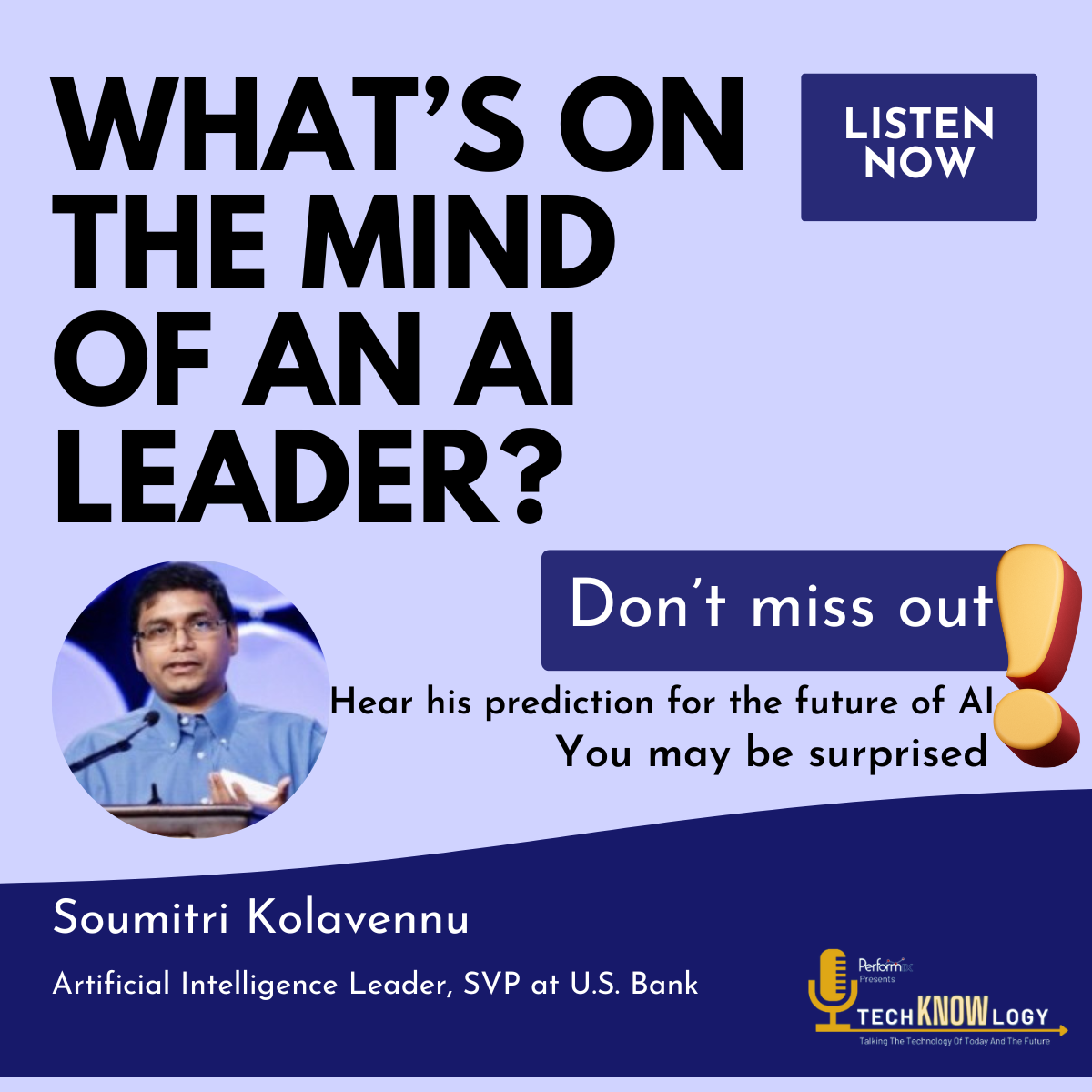
New Podcast
Soumitri Kolavennu, the visionary AI leader at U.S. Bank, is spearheading a revolution that unlocks the full potential of AI dominance and unleashes unstoppable innovation.
Streaming Now on Spotify
Generative AI in Manufacturing: Real-World Success Stories
Unlocking the potential of generative AI, manufacturing giants are reshaping production processes with innovative implementations. Here are compelling case studies showcasing the transformative impact of generative AI in the manufacturing sector:
1. Mahindra & Mahindra (M&M): Virtual Assistants for Swift Issue
Resolution
- Objective: Reduce machine downtime and expedite issue resolution.
- Implementation: M&M deployed generative AI-powered virtual assistants for its production teams. By aggregating technical data and maintenance records, the Virtual Assistant addresses technical issues swiftly. It interprets error codes, providing step-by-step instructions to minimize downtime.
2. BMW Group: Quantum: Inspired Generative Model for Plant Scheduling
- Objective: Optimize plant scheduling to minimize assembly line idle time.
- Implementation: BMW utilized a Quantum-Inspired Generative Model, GEO, for plant scheduling. Classic optimization solvers generate schedules refined by GEO's quantum-inspired models. The result? Efficient plans surpass traditional solvers, minimizing idle time while meeting production targets.
3. Hitachi: Skills Transfer with Generative AI in Training
- Objective: Mitigate the impact of mass retirements by transferring expert skills to younger workers.
- Implementation: Hitachi employed generative AI to create immersive training experiences. The system generated training videos depicting real-life scenarios in maintenance and manufacturing, providing valuable skills transfer to the younger workforce.
4. Merck: Synthetic Images for Defect Detection
- Objective: Overcome the challenge of limited training data for AI and ML models in defect detection.
- Implementation: Merck's AI engineers used generative AI techniques, including GANs and Variational Autoencoders, to create synthetic defect image data. These synthetic images significantly improved the accuracy of defect detection models, reducing time-to-market for new models.
Read the Complete case study of Generative AI Solutions at Merck's
5. Sereact: Vision for Warehouse Robots through Generative AI
- Objective: Enable robots to perform "pick-and-pack" tasks in warehouse operations autonomously.
- Implementation: Sereact revolutionized warehouse operations with a transformer model trained on billions of simulated images. The model, interacting with a language model, allows operators to input text commands through a chat interface, streamlining robot task planning.
Conclusion: Navigating the Future of Manufacturing with Generative AI
Generative AI is not just a fleeting trend; it has firmly established itself as a permanent force reshaping the manufacturing landscape. Its impact spans from minimizing downtime and optimizing schedules to overcoming data limitations and empowering robot autonomy, showcasing its diverse applications. Succeeding in the implementation of generative AI requires a strategic approach: identifying the right use cases based on AI maturity, initiating small-scale projects, and gradually expanding. In navigating this era of AI-driven manufacturing, manufacturers can achieve innovation and excellence by prioritizing business impact, AI readiness, and data readiness in their generative AI initiatives.

Far from a mere technological buzz, generative AI is silently spearheading a revolution in manufacturing. According to McKinsey, its potential contributions could reach USD 13.5 trillion by 2030. The manufacturing sector, encompassing automotive, aerospace, pharmaceuticals, and semiconductors, is poised to capture a substantial value ranging between USD 270 billion to USD 460 billion. This underscores not only the transformative power of generative AI but also the immense economic opportunities it presents for forward-thinking manufacturers.